
Shipping an AI feature in 2025 is easy. Building an AI product that users adopt, trust, and return to consistently is another challenge entirely.
At Polyform, we partner with AI founders and product teams pushing beyond basic implementations to create human-centered AI tools. One pattern is clear: the primary cause of drop-off in AI products is not model quality. It is interaction design.
If you are serious about retention, it is time to design beyond the chatbot.
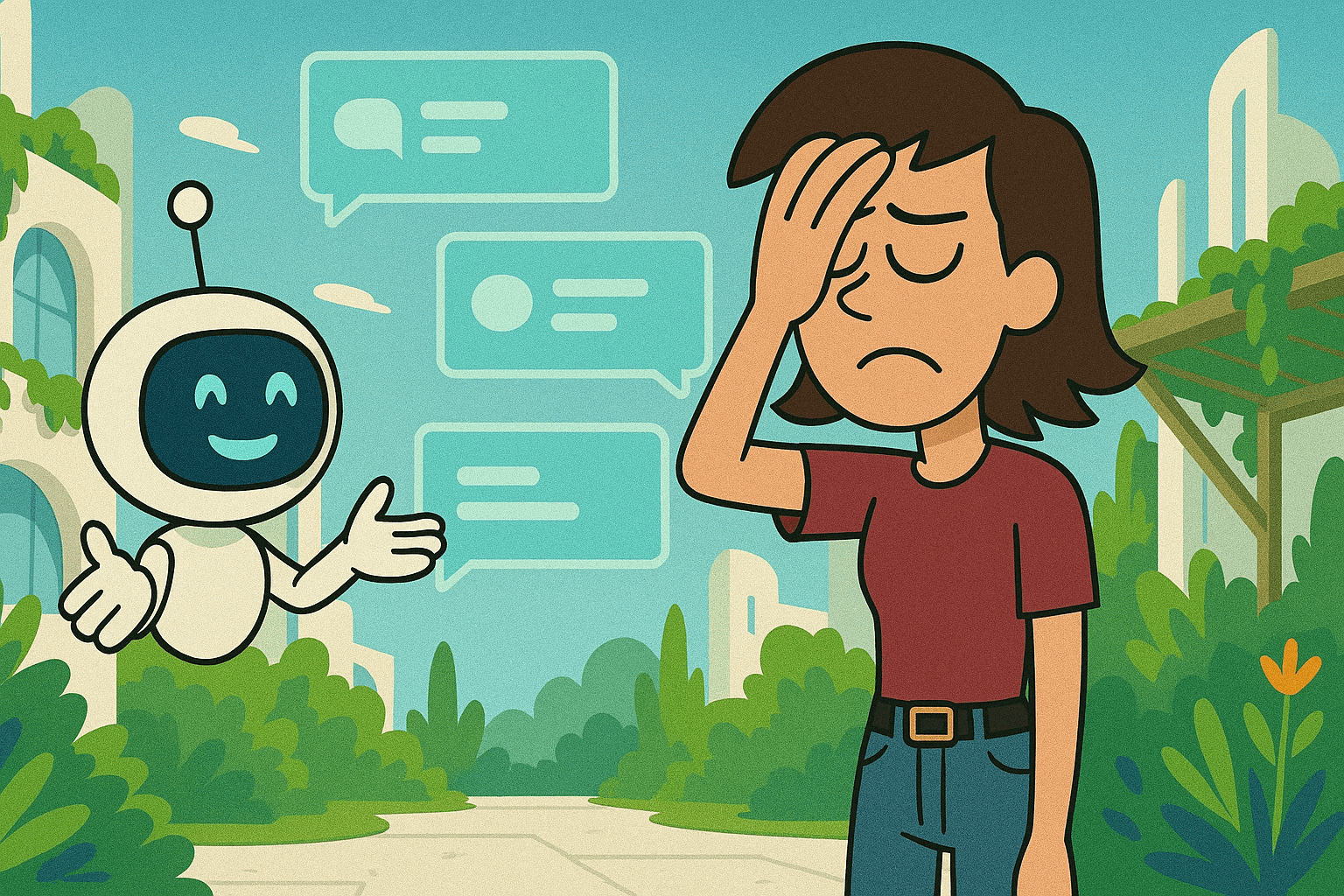
Why Traditional Chatbot UX Underperforms
Chatbots were a natural first container for LLMs. Free-form text inputs offered flexibility.
But that flexibility often leads to friction in real-world use:
- Users are unsure what queries are possible or effective.
- Open-ended prompting creates cognitive overload.
- Conversation threads feel linear and inert rather than collaborative.
- Lack of system transparency erodes user trust after initial interactions.
Chatbot UX is often too unstructured, too passive, and too unpredictable to sustain engagement at scale.
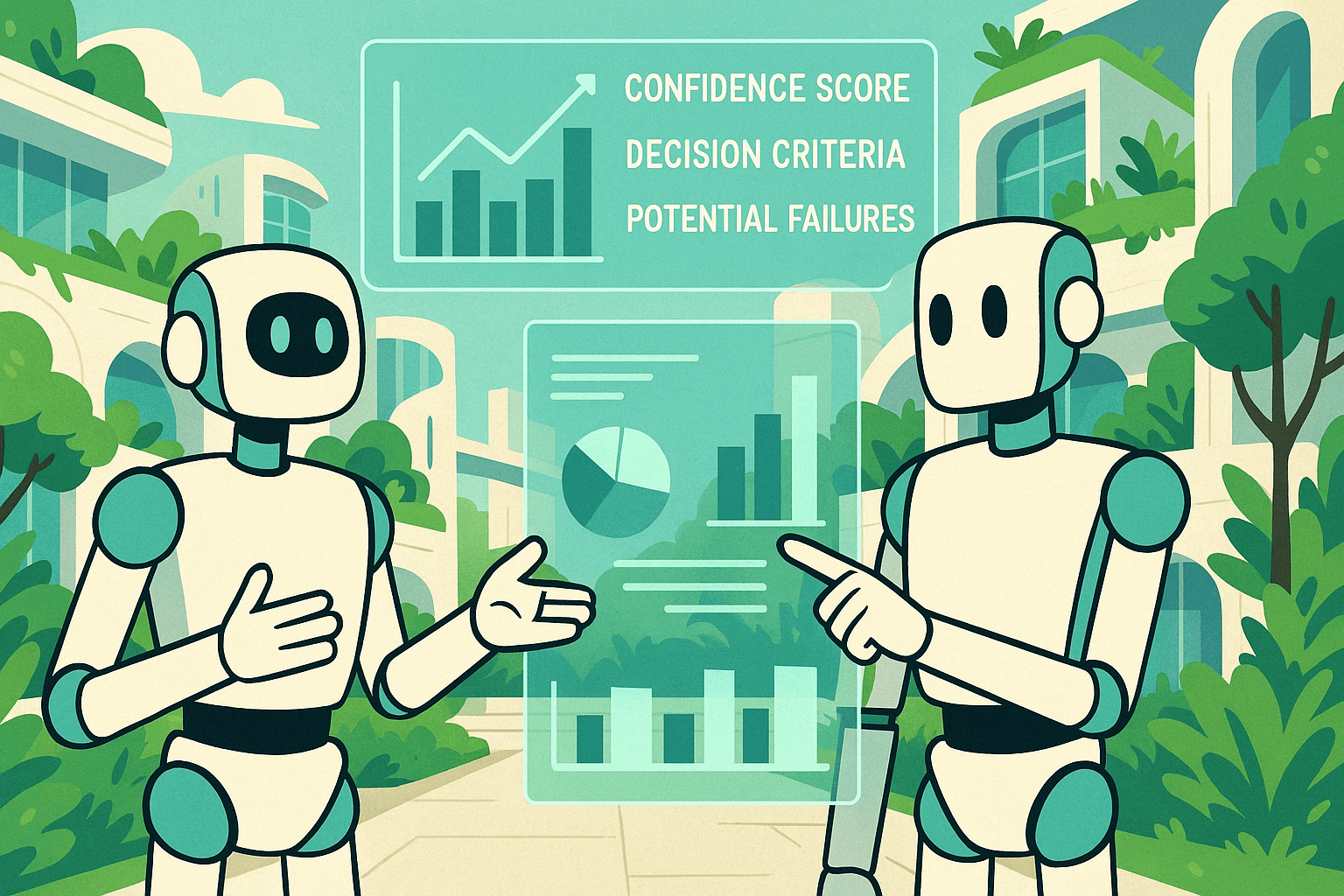
AI as Collaborative Tool, Not Conversational Toy
Successful AI products position the model as an internal engine supporting structured, user-driven workflows, not as the primary UI.
Key design principles include:
- Guided Intent Capture: Use dynamic prompt builders, modular input fields, and contextual UI hints to reduce user uncertainty and guide interaction.
- Progressive Disclosure of AI Output: Surface draft responses, ranked options, or iterative suggestions rather than demanding full free-form queries.
- Editable, Modular Outputs: Enable granular refinements through in-line editing, selective regeneration, and parameter tweaking.
- Transparent System Behavior: Clearly communicate AI confidence scores, decision criteria, and potential failure modes.
These approaches shift AI products from passive answer generators to active problem-solving assistants.
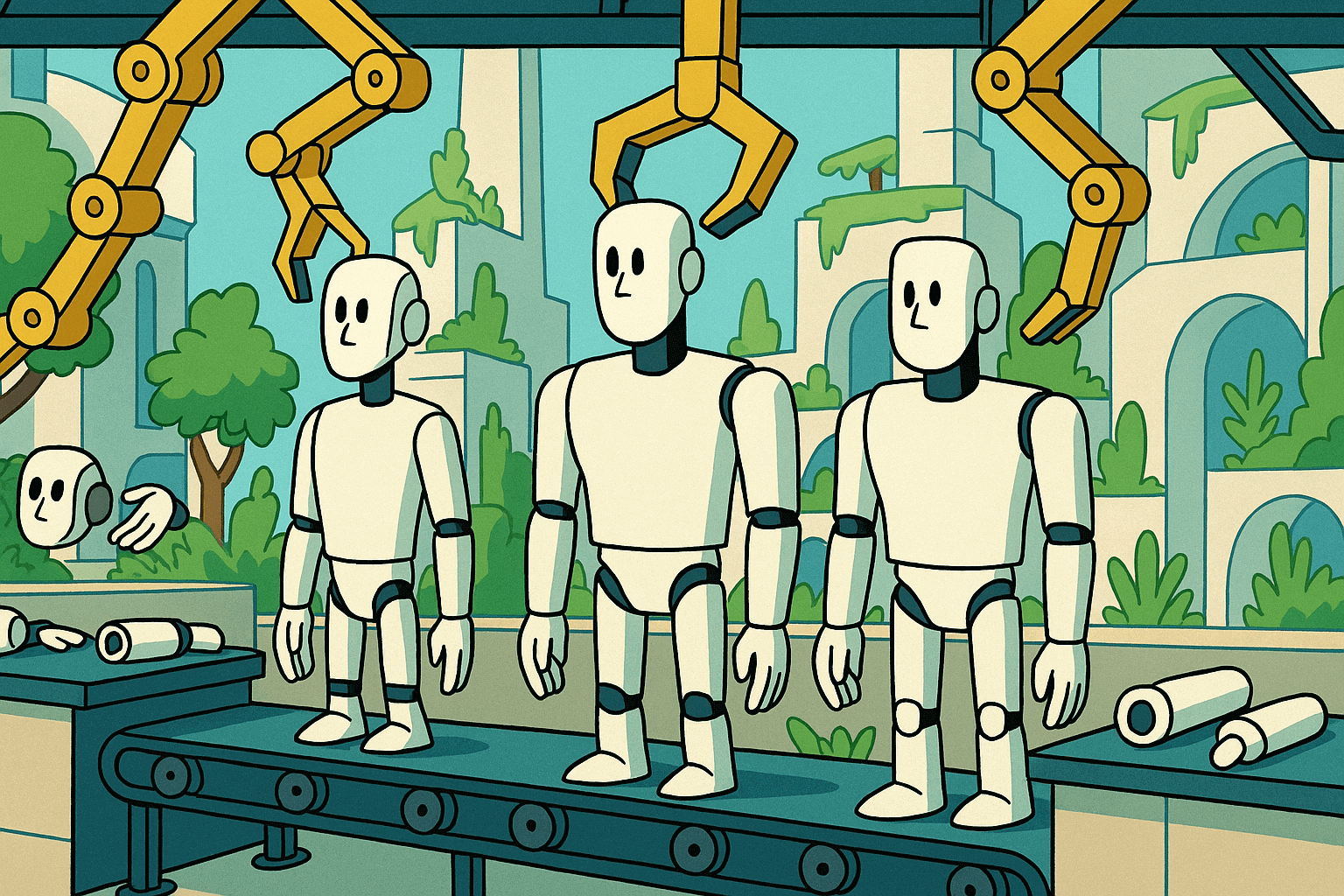
Proven UX Patterns for High-Retention AI Products
Interaction models that consistently outperform chatbot paradigms include:
Co-Authoring Workspaces: AI proposes drafts, users refine iteratively, system learns adaptively.
Smart Workflow Templates: Pre-scaffolded task flows that the AI populates or adapts dynamically.
Contextual Copilots: AI operates in the background of user workflows, offering interventions based on real-time context.
Action Suggestion Interfaces: Predictive, high-confidence micro-actions offered at relevant touchpoints.
Each pattern prioritizes minimizing cognitive load, accelerating intent capture, and maintaining user agency.
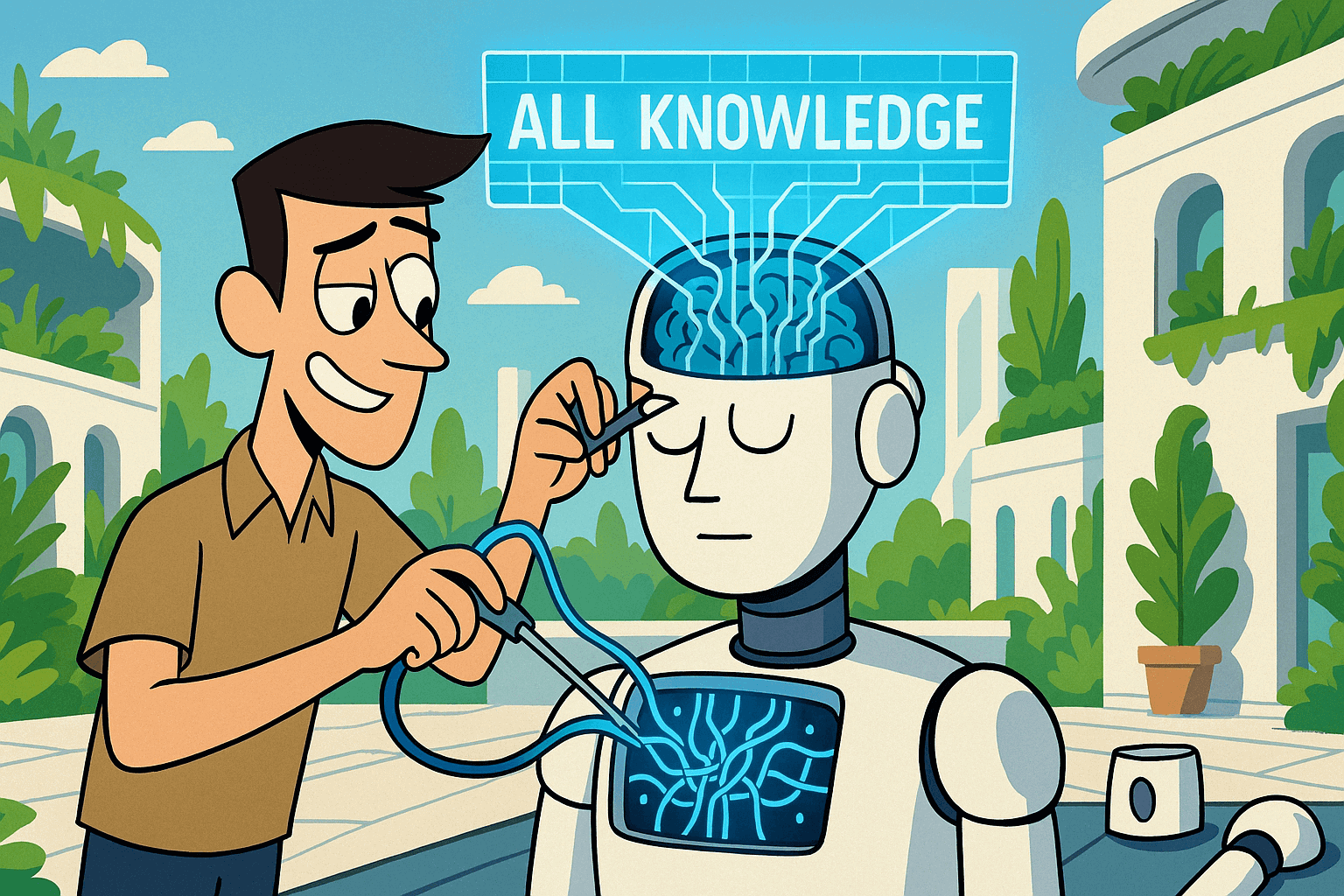
Designing Retention Mechanisms into AI Tools
High-retention AI UX is built on consistent value delivery, adaptive personalization, and visible user control.
Retention strategies embedded from day one include:
Personalization Layers: User preferences and interaction history dynamically shape future system behavior.
Memory Surfaces: Expose editable system memory constructs so users can curate what the AI knows.
Micro-Feedback Channels: Lightweight mechanisms for users to correct, rate, or redirect AI outputs in session.
Multi-Modal Interaction Paths: Seamless transitions between typing, selecting, speaking, and editing to suit user context and preference.
Effective retention in AI products stems from designing ongoing trust loops, not one-off novelty.
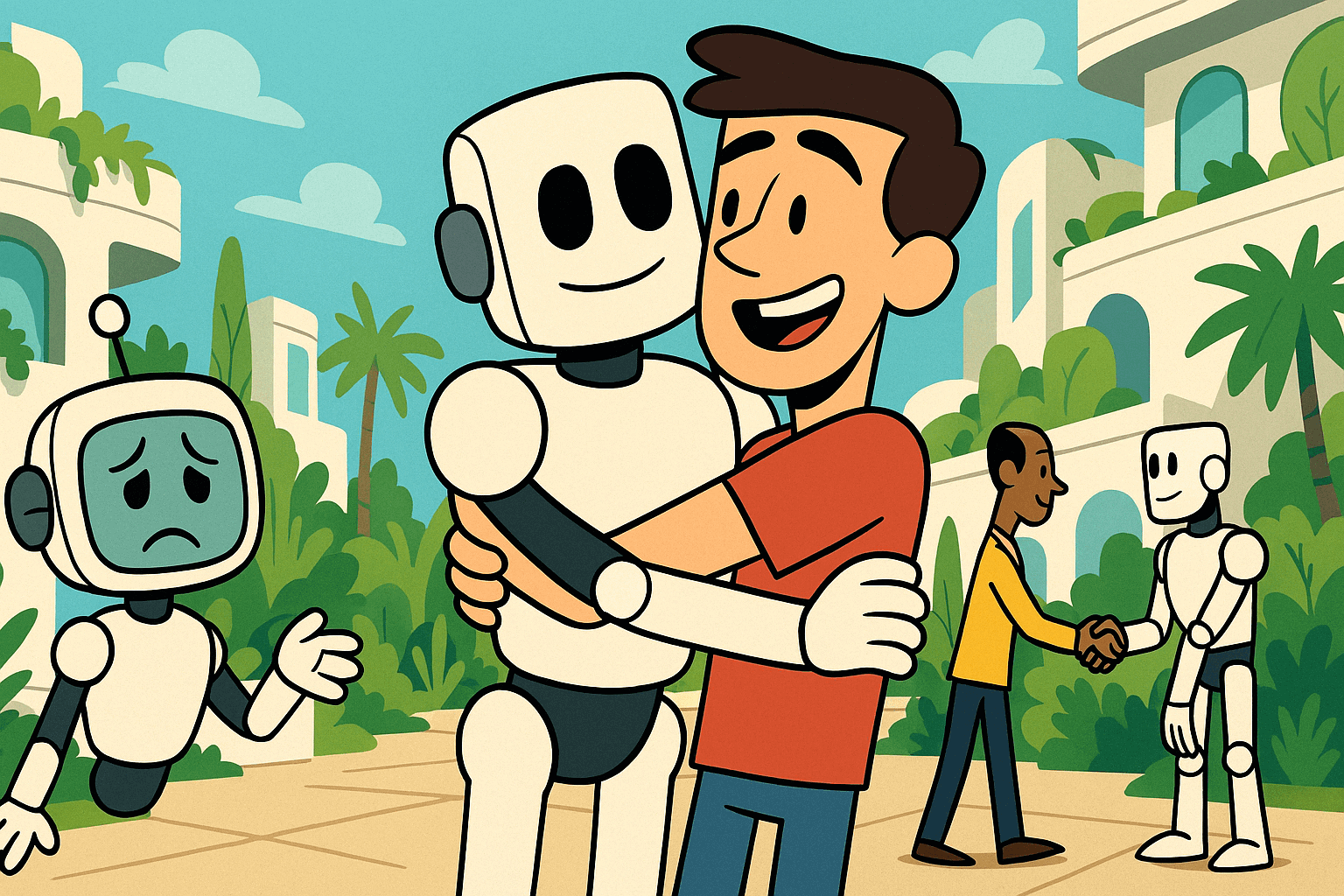
Conclusion: The Future of AI UX is Cooperative, Not Conversational
Basic chatbots are insufficient for building durable AI products. True success will come from designing cooperative systems where AI augments human capabilities transparently and adaptively.
At Polyform, we specialize in transforming cutting-edge AI into practical, scalable products built for real users. If you are ready to move beyond the chatbot phase and design AI tools that users actually rely on, we are ready to build with you.
Thanks for joining us!
Now check your spam folder to secure your newsletter
More thoughts
Hire Polyform and bring the power of design to your startup.
Collaborate with Polyform to bring your big idea to market faster, better, & stronger.